News
Discover latest research in drug development including updates on our collaboration with the FDA and patents for our novel image-based analysis.

Thank you! Your submission has been received!
Oops! Something went wrong while submitting the form.
Transform Your Program with Microstructure Science
Get started with a drug product digital twin.
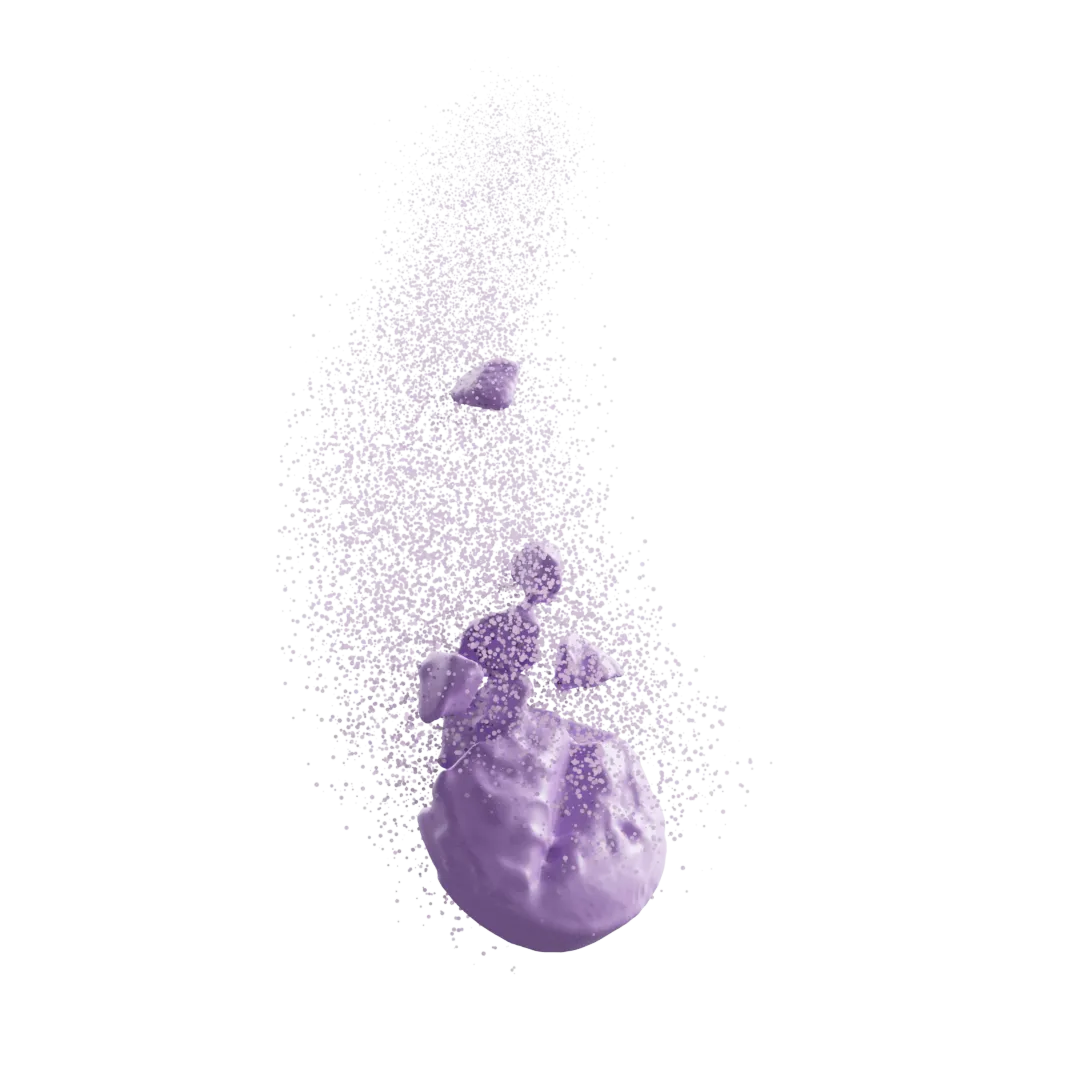