Deriving Powder Mixing Indices from AI Analytics

Advanced image analytics to study powder mixing in a novel laboratory scale agitated filter dryer
Powder mixing unit operations are essential to the manufacturing of pharmaceutical drug substance and drug products. To model powder mixing, computational approaches have been used to evaluate mixing indices for different types of mixers, while also necessitating experimental methods for tracking of particle dynamics for validation. In this work, the experimental mixing performance of two particle species of different particle size and densities in a novel laboratory agitator filter dryer (AFD) was evaluated by performing 3D X-ray microCT (μCT) analysis. A new workflow, in which the μCT images were pre-processed with image analytics of increasing complexity and fidelity from image thresholding to advanced AI-based image segmentation and 3D reconstruction, was demonstrated to derive powder mixing indices. The AFD device which enables a customizable laboratory equipment for scale-down experimentation of agitated drying was shown to be capable of achieving a uniform powder mixture with micromixed cluster domain size.

Raimundo Ho, Yujin Shin, Shawn Zhang, Aiden Zhu, Prashant Kumar, Himanshu Goyal
Published with AbbVie and Indian Institute of Technology Madras
https://doi.org/10.1016/j.powtec.2023.118273
Additional Publications
Transform Your Program with Microstructure Science
Get started with a drug product digital twin.
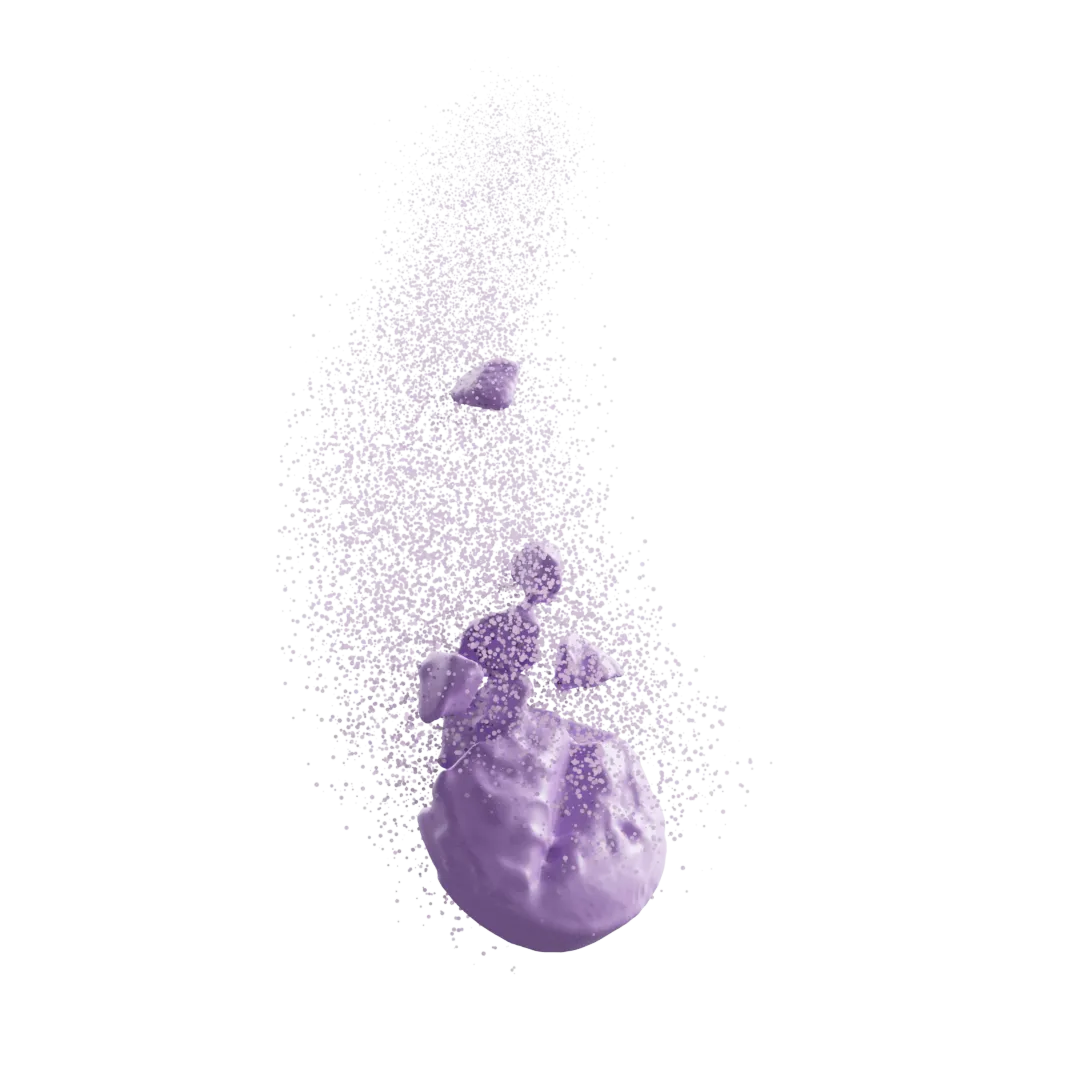